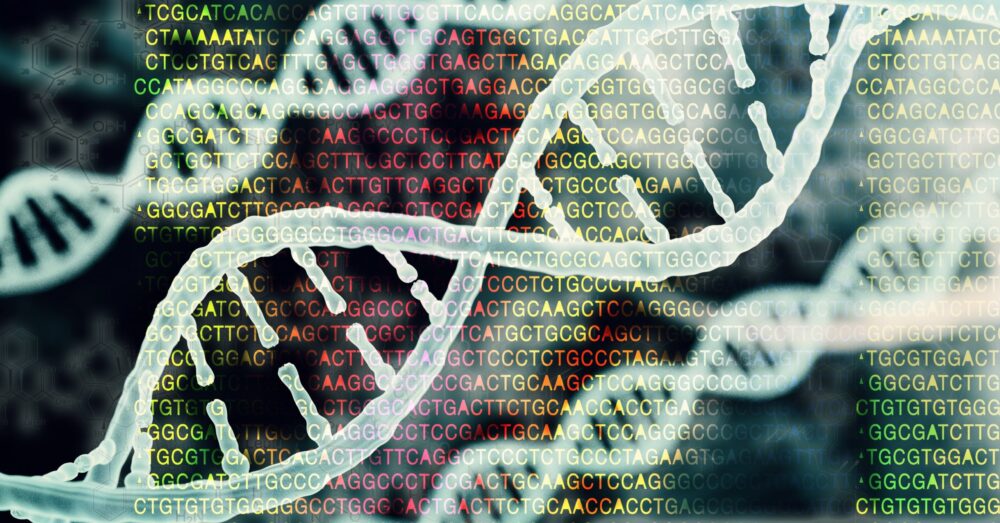
The emerging era of pharmacogenomics
Sir Archibald Garrod, a British physician, first discussed his concept of ‘chemical individuality’ in The Inborn Errors of Metabolism, where he writes, “Every active drug is a poison, when taken in large enough doses; and in some subjects, a dose which is innocuous to the majority of people has toxic effects, whereas others show exceptional tolerance of the same drug.”1
This observation on the heterogeneous nature of human drug response made over a century ago was the pioneering principle that has led to the field of pharmacogenomics (PGx), where we now recognize that human genetic variation can influence an individual’s response to drug.
Today, close to 15% of EU-EMA and US-FDA approved medications have PGx information in their labels2. The Clinical Pharmacogenetics Implementation Consortium (CPIC), established in 2009, publishes guidelines to help clinicians understand how to use genomic information to inform drug prescription, including a standard system for graded levels of evidence linking genotypes to phenotypes, to facilitate the translation of pharmacogenomic knowledge from bench to bedside3.
With the advances in genotyping technology and NGS, clinical laboratories are starting to transition from single-gene tests to multi-gene panels for PGx, with SNV panel testing still being the most widely used technology. The SNV data from patients must first be translated into predicted drug metabolizer phenotypes; this interpretation can be relatively straightforward or challenging depending on the number of variants and haplotypes present in translation guidelines for that drug-gene pairing4. The complexity of computational approaches involved in variant interpretation hence typically requires a substantial level of expertise and manual input. As Relling and Evans observes in a review published in Nature, the cost of implementing pharmacogenomics in a clinical setting is “shifting from the cost of the laboratory test to the costs associated with linking genetic test results with evidence-based decisions that will robustly guide prescribing”.5
Translating pharmacogenomics into clinical decisions
Dr Goh Liuh Ling and her team at Tan Tock Seng Hospital, one of the largest public hospitals in Singapore, face these challenges daily. As the director for the Molecular Diagnostic Laboratory (MDL), Dr Goh is responsible for implementing clinical genomics workflows and ensuring that patient clinical reports are generated in an accurate and timely manner. Her lab uses the Applied Biosystems® QuantStudio™ 6 Flex Real-Time PCR System, TaqMan genotyping software, and AlleleTyper software for PGx testing. Dr Goh was interested in scaling up her lab’s sample management and PGx workflow to accommodate increasing clinical demand and to meet the needs of the future, but she realized that they were constrained by the fragmented and highly manual workflow for genetic data production and report generation. This process involved many steps requiring manual curation and multiple lab personnel verifying the results, such that the time spent generating one report from a multi-gene panel took close to 30 minutes, and the overall turnaround time from sample receipt to report generated would typically take 10 days.
“Using BC|GENOME for automated data processing and interpretation,
there was a 6-fold reduction in the time spent on generating a single clinical report”
With the intention of future-proofing the analytics infrastructure in her lab, Dr Goh decided to evaluate a clinical solution provided by BC Platforms (BCP) for sample data management, automated data processing and interpretation. BC|GENOME is a CE-IVD certified data management and analysis solution that provides a streamlined user experience and can be integrated into existing LIMS and EHR systems. Dr Goh’s team, including Chia Wei Lim, a genetic counsellor at MDL, worked closely with Ratika Sianturi, a Senior Customer Success Engineer at BCP, to implement the holistic solution stack according to the lab’s specifications for managing samples, creating orders, registering ThermoFisher sample worksheets and processing genotype results from the ThermoFisher system. Additionally, the AlleleTyper output was integrated with MDL’s in-house PGx reference database and drug recommendations for custom report generation. A summary of the end-to-end PGx workflow installed at MDL is shown in Figure 1, from sample input to clinical report.
Using this automated PGx testing pipeline, the MDL team found that there was a 6-fold reduction in the time spent on generating a single clinical report, cutting down the time required from 30 minutes to 5 minutes. Correspondingly, the turnaround time for returning a PGx report to the patient could be reduced from 10 to 5 days, providing timely guidance to clinicians making prescribing decisions. As a result of utilizing BC|GENOME for PGx reporting, the automatic data processing helped MDL to reduce the number of man-hours required for variant curation and interpretation, minimized the chance for human errors including typos, and ultimately helped Tan Tock Seng Hospital to improve patient care with a shorter turnaround time for PGx testing.
Figure 1. BC|GENOME provides a streamlined end-to-end solution for sample data management, batch processing, and report generation.
The need for wider implementation of pharmacogenomic testing
As research in the field of PGx continues to advance, an increasing number of healthcare institutions are moving from utilizing PGx reactively i.e., ordering a test when there is a need to prescribe a high-risk drug, to preemptive PGx testing, such that broad screening of multiple pharmacogenes and recommendations for dosing are readily available in patient electronic health records.6 Indeed, there are several ongoing projects in the US and Europe trialing this concept, such as the Electronic Medical Records and Genomics PGx project (eMERGE-PGx), initiated in ten US medical centres and implementing PGx testing for 84 proposed pharmacogenes in 9000 patients, and the Ubiquitous Pharmacogenomics Consortium (U-PGx) is implementing and evaluating the impact of PGx testing on therapy outcomes using more than 40 clinically relevant PGx markers in seven European clinical centers.7
These large-scale PGx initiatives will undoubtedly help to improve patient outcomes, reducing the incidence rate of adverse drug reactions and the associated economic burden on healthcare systems, addressing a well-recognized public health issue in countries all around the world.8–11 However, the successful implementation of widespread PGx testing critically hinges on clinical laboratories being able to streamline the reporting process and overcome hurdles with variant interpretation. At least for Dr Goh, she knows that MDL is now ready to meet these challenges of the future with BC Platforms technology.
References
- Garrod, A. The inborn factors in disease. Southern Medical Journal 24, (1931).
- Ehmann, F. et al. Pharmacogenomic information in drug labels: European Medicines Agency perspective. The Pharmacogenomics Journal 15, (2015).
- Relling, M. v & Klein, T. E. CPIC: Clinical Pharmacogenetics Implementation Consortium of the Pharmacogenomics Research Network. Clinical Pharmacology & Therapeutics 89, (2011).
- van der Lee, M., Kriek, M., Guchelaar, H.-J. & Swen, J. J. Technologies for Pharmacogenomics: A Review. Genes 11, (2020).
- Relling, M. v. & Evans, W. E. Pharmacogenomics in the clinic. Nature 526, (2015).
- Krebs, K. & Milani, L. Translating pharmacogenomics into clinical decisions: do not let the perfect be the enemy of the good. Human Genomics 13, (2019).
- van der Wouden, C. H. et al. A brighter future for the implementation of pharmacogenomic testing. European Journal of Human Genetics 24, (2016).
- Qing-ping, S. et al. Consequences, measurement, and evaluation of the costs associated with adverse drug reactions among hospitalized patients in China. BMC Health Services Research 14, (2014).
- Plumpton, C. O., Roberts, D., Pirmohamed, M. & Hughes, D. A. A Systematic Review of Economic Evaluations of Pharmacogenetic Testing for Prevention of Adverse Drug Reactions. PharmacoEconomics 34, (2016).
- Formica, D. et al. The economic burden of preventable adverse drug reactions: a systematic review of observational studies. Expert Opinion on Drug Safety 17, (2018).
- Chan, S. L. et al. Economic burden of adverse drug reactions and potential for pharmacogenomic testing in Singaporean adults. The Pharmacogenomics Journal 19, (2019).