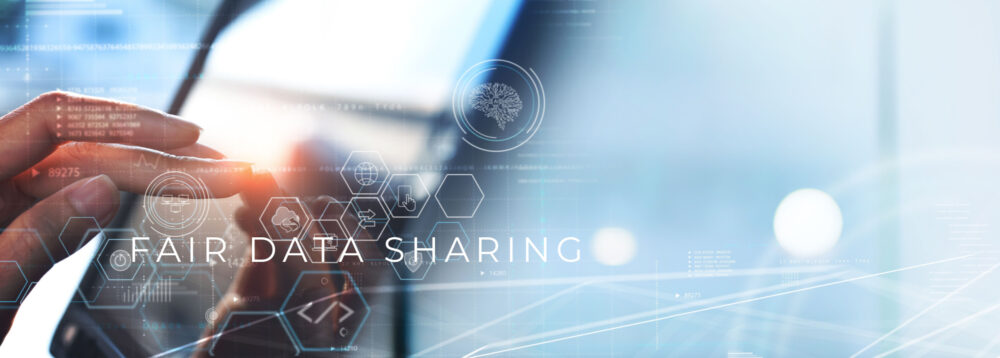
The FAIR data principles – making research data Findable, Accessible, Interoperable, and Reusable – are an important framework guiding efforts to improve data management and sharing in the life sciences. Properly implementing FAIR has the potential to accelerate discovery, enable new integrative analyses, reduce duplicated efforts, and promote open and reproducible science. However, realizing this potential in practice faces a myriad of technical, ethical, motivational, and infrastructure challenges.
A survey on data sharing attitudes found 90% of life scientists support FAIR principles, but only 22% applied them in practice (Ali et al., 2020). This gap highlights hurdles still needing community-wide effort. Challenges include lack of standards, privacy/ethics concerns, proprietary data interests, outdated databases, few incentives, and cultural inertia against open data (Samur et al., 2021). But the tide is turning, with growing policies and initiatives aimed at facilitating and rewarding FAIR data sharing.
Funders like the NIH, Wellcome Trust and Chan Zuckerberg Initiative now require FAIR data management plans for grants (Hodson et al., 2021). The NIH STRIDES initiative is developing ontologies, identifiers and standards to enhance biomedical data FAIRness (Sansone et al., 2019). Publishers are increasingly mandating open data availability for publication, with some requiring FAIR principles (Cousijn et al., 2021). Repositories are collaborating on federated systems, like NIH’s AnVIL, to enable unified analyses across datasets (Raj et al., 2020). And large pharma companies like GSK are voluntarily releasing clinical trial data in machine-readable formats (Norgeot et al., 2020).
Beyond top-down policies, community efforts such as GO FAIR are providing implementation guides, tools, and training to address FAIR adoption challenges (Jacobsen et al., 2020). The ELIXIR FAIRplus project helps train researchers on FAIR data stewardship and provides templates for data management plans (Lin et al., 2021). The FAIRsharing registry indexes standards, repositories, and policies to improve findability of resources (Sansone et al., 2019). And the open source CEDAR metamodel enables semantically annotating and integrating biomedical data (Yu et al., 2020).
These initiatives demonstrate that, while challenging, concerted efforts across stakeholders can systematically address barriers to FAIR data sharing. But continued progress requires focused work, including:
- Developing standardized ontologies, identifiers, and semantic annotations tailored for biomedicine data (Yu et al., 2020).
- Federation of repositories into searchable data commons, while maintaining data protections (Raj et al., 2020).
- Publisher enforcement of open data policies, with FAIR requirements (Cousijn et al., 2021).
- Funders providing infrastructure and incentives for FAIR data curation and sharing (Hodson et al., 2021).
- Showcasing benefits of FAIR sharing through use cases and success stories (Jacobsen et al., 2020).
- Training researchers on FAIR skills, and meaningful cultural changes (Lin et al., 2021).
The life sciences community is recognizing that the benefits of FAIR data sharing outweigh the costs. Through continued collaboration, development of tools and standards, and implementation of supportive policies, the goal of FAIR data driving discovery can be translated into reality. But this requires deliberate, long-term effort to reshape practices and norms around open sharing and responsible reuse of biomedical data.