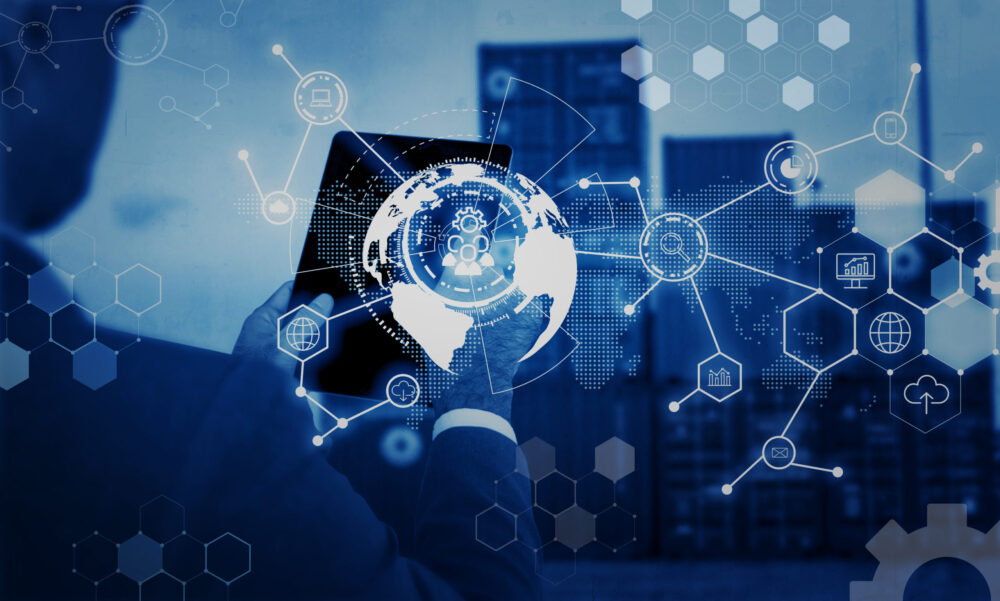
Exponential growth in available data and the associated challenges
In recent years, health systems have been generating more and more data. Health records have been transitioning to a digital format and standards for storing and handling such data have been rapidly developing. The growth in healthcare data volumes is driven by an increased need for personalized medicine. Adoption of personalised and preventive medicine practices have the potential to significantly reduce costs of healthcare while improving its quality. In order to achieve personalized care, large amounts of data are collected, considering a comprehensive patient profile with ethnicity, lifestyle, environmental and biological factors.
This shift to personalized healthcare has also created increased pressure in the pharma industry to develop better therapeutics, with higher return on R&D investments and shorter timelines. Due to this transition, there has been increased adoption of RWD in pharma, which in turn has created a need for access to a wider and more diverse pool of patient data. This data holds increasing value in shortening R&D timelines, helping avoid adverse events, and bringing deeper insights into underlying disease biology.
Though incentives for use are clear, accessing large and diverse datasets – also including genomic data – remains a challenge. The lack of fast access is a significant hurdle to unlocking the data’s full value. Data is scattered, siloed, and often structured in various formats that make it more difficult to use in combination. Additionally, divergent access governance and complex legal and ethical framework create additional requirements. Concerns surrounding data access control have led to tightened privacy legislations, as we have seen with the EU General Data Protection Regulation (GDPR), the California Consumer Privacy Act (CCPA), and the Health Insurance Portability and Accountability Act (HIPAA) of the United States.
Additionally, these regulations are predicted to continue growing globally as more data is collected. At the moment, only around 10% of the world’s population is protected by the GDPR or similar laws, but Gartner Research predicts that this will be around 50% by 2022.
Safe Data Sharing with a Federated Network Approach
To address the numerous challenges with secure data access, federated technology is a promising solution. This approach allows for safe data sharing with controlled access to diversely regulated data with minimised risk. With this approach, researchers can access data for analysis across institutions without sharing any identifying information, as the individual-level data never leaves its institution of origin. In a federated network, participating institutions may not even know the other members, and the original data ownership is maintained.
Anni Ahonen-Bishopp, Solution Director of Pharma and Research at BC Platforms, says the immediate benefit of Federated Learning is the quick access to data. “A federation model can be applied to various different questions on various levels and that gives pharma companies and CRO’s much more diverse data, much faster.”
The current paradigm for multi-institutional collaboration in healthcare requires institutions share patient data to a centralized location. This is often called collaborative data sharing (CDS). However, according to the 2020 nature.com Scientific Report “Federated learning in medicine: facilitating multi-institutional collaborations without sharing patient data,” CDS models have a hard time generalizing their data to external institutions, or even within institutions. CDS also does not work well with large numbers of collaborators, domestic or international, due to privacy and data ownership concerns.
In contrast, federation allows for a collaborative learning method where multi-institution collaborators can examine the data and perform analyses such as machine learning (ML) algorithms on the data. This approach gives researchers in an increasing number of cases as much freedom in analysis methods as traditional data sharing, but it is much more collaborative and the data remains in one location, increasing efficiency and maintaining security. In the case of AI/ML tools, for example, researchers send their updates to a central server to be aggregated into a consensus model. The aggregation server sends this model to collaborating institutions for them to use and train further. Therefore, with a federated data sharing approach, there is less room for error and the timelines are shortened for the return of results. It enables a certain openness and flexibility which allows for more learnings and good quality insights from the data. When it comes to generalizability, federated learning models among 10 institutions can have as much as 99% of the model quality of similar research with CDS. As when using FL it is easier to get access to a larger number of data collections than with CDS, in practise FL models are usually superior compared to CDS.
As more institutions adopt FL, shared models may also grow on datasets of unprecedented size. This will have a great impact on personalized medicine as data diversifies. Says, Timo Kanninen, CSO and founder of BC Platforms, “The amount and diversity of data are really the keys. Especially with genomics, diversity is very important.”
Conclusion
A federated approach to data sharing facilitates large-scale multi-institutional data learning, while also following data protection regulations of GDPR, CCPA, and HIPAA. As these regulations increase and the need for diverse data grows, innovative data sharing methods like federation may become the only way to access data securely and efficiently.
Says Kanninen, “We see two trends— the use of AI models in healthcare, and increasingly strict data regulations. Federated AI will be big in the very near future.”